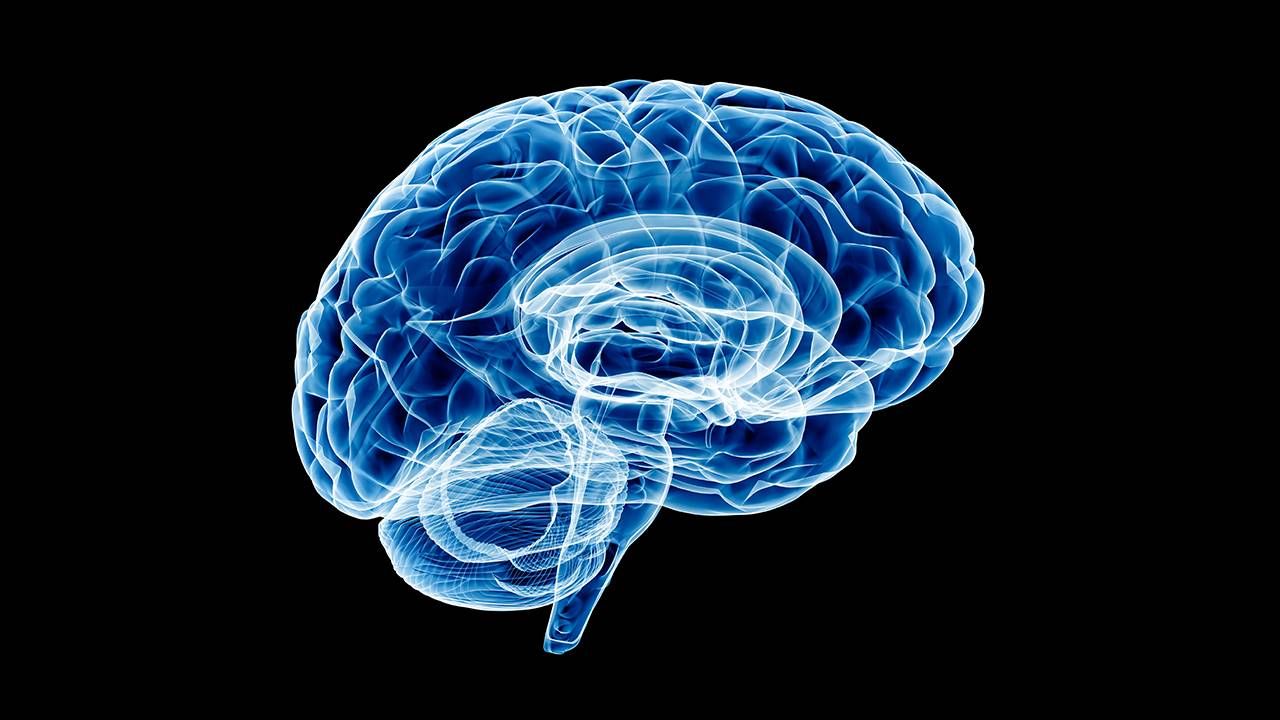
What We Now Know About 'Brain Age' and Dementia
By The American Federation for Aging Research (AFAR) for Next Avenue
Dr. Brandon Westover's training points to his passion for the brain, mathematics and computing. He holds a Ph.D in physics and is a neurologist with specialty training in epilepsy and clinical neurophysiology; his research has been bolstered by a 2018 Breakthroughs in Gerontology Award from the Glenn Foundation for Medical Research and the American Federation for Aging Research. An associate professor of neurology, Massachusetts General Hospital and Harvard Medical School, he runs the inpatient critical care brain monitoring service at Massachusetts General Hospital, which sparked his research interest in sleep.
In this interview, Westover explains how his research explores abnormal sleep patterns and how to improve sleep for better cognitive outcomes.
Why is sleep particularly important as we age?
Sleep is important for development, but also for healthy aging. As you get older and you're more at risk for problems with cognitive speed or other cognitive functions like memory, protecting your brain against the wear and tear of age becomes more and more important.
There are a couple of things that we've learned relatively recently about sleep that are still being actively researched.
First, sleep helps clear out the garbage that your brain makes in the daytime. There's this thing called the glymphatic system, which is the brain's way of flushing out the byproducts of everything the brain does during the daytime while we are awake. During the daytime, cells are working and are actually a bit larger than when you go to sleep. When you sleep, the space between the cells opens ups and it allows for this clearance mechanism to drain things out like proteins and other waste produce that accumulate during the day when cells are working.
Sleep also helps prepare and hold onto memories.
The deepest stage of sleep is where most of the garbage clearing happens.
There's an intermediate stage of sleep called non-REM Stage 2. This has functions related to memory consolidation. There are these beautiful waves on EEG [an electroencephalogram brain test] that occur in that part of sleep called sleep spindles. These sleep spindles facilitate taking the short-term storage of your brain's inbox and putting the information into long-term storage in the cortex of the brain.
Then, there is REM sleep. REM is a qualitatively different part of sleep where most of the vivid sort of dreaming occurs. That's thought to be important for forming associations between memories and ideas, among other things.
How has AI [artificial intelligence] changed how sleep analysis is done clinically and commercially?
Sleep is analyzed clinically by visual analysis. Experienced technicians and sleep specialists score sleep studies by hand and then make a diagnosis based on that.
It's easy to underestimate how difficult it is to beat what humans do, so many commercial sleep studies claiming to do this are unfortunately mostly hype. They've often solved the wrong problem or an overly simplified version of the problem, usually by focusing on relatively small numbers of people and detecting sleep problems in data from relatively healthy people or people without neurologic disease.
Commercially, we're not where we should be before we can rely on AI to replace what sleep specialists do. However, in the research realm, we are largely in that position right now.
We can do sleep staging, for example, better than people can — faster and more reproducibly. Actually, when you look at differences between humans and AI algorithm scores trained on appropriately diverse sets of patients, usually the AI algorithm is right. So even though humans are the gold standard right now, it turns out that properly trained AI is usually right.
The more exciting thing is that it's possible to go well beyond what humans can do because the way that a lot of the patterns in sleep data were defined in the first place is based on educated guesses rather than a data-driven sort of process.
To explain this, I like to contrast two kinds of AI:
- One type of AI uses annotated data that humans have scored or labeled and tries to reproduce human behavior, or even better, the average of a group of experts rather than the behavior of one individual. This averages out idiosyncrasies or personal differences but is still ultimately reproducing something based on educated guesswork and simple pattern recognition.
- I like to call the other way “outcome-driven AI.” The idea is that whatever sleep quality is, it shouldn’t just be whatever “looks good” or normal to us. Instead, it should be what patterns in the data allow reliable predictions of outcomes like risk of heart attack, stroke or dementia.
That outcome is the target and then you design an algorithm that learns how to pick out the things that are different about people who ended up having those problems from the ones who remain healthy. This approach is not being routinely used clinically and, to my knowledge, not available in commercial products, but our research is on the cusp of changing that.
How is sleep data used in the Sleep Brain Aging Index (BAI)?
The sleep brain age index is similar to an idea that has been used in MRI research.
In the MRI research world on "brain age," first, you take a whole bunch of MRIs from relatively healthy individuals at different ages and ask: What are the typical structural characteristics of a healthy brain? For example, how large is the brain; how large are the different parts; how thick are the layers of the brain.
Then, you compare characteristics of a new individual's brain MRI with characteristics typical for each age and pick the age that matches best. The difference between that age and the person's actual age is the brain age index. If the age is bigger than the person's actual age, then they would have an elevated BAI or MRI brain age index. Which means that their brain "looks" like older than a person's chronological age.
It's the same idea with sleep data. We take measurements of brain activity non-invasively with EEG electrodes on the scalp, collect data over the course of a night of sleep and then we automatically stage it.
From each of the stages, we pick out a series of features that characterize the sleep. Then, we compare those with what's normal for each age. Then we say, "Okay, this sleep looks like someone who's 60." If your age is 40, then that may be a concern. Or, if your sleep looks like you are 50, maybe that's a good thing. We call the difference between "brain age" and chronological age the brain age index, or BAI.
We've found that BAI does turn out to be a good signal for brain health.
We've recently published findings about patients with chronic HIV: although treatments are quite good now in terms of preventing progression of HIV, a lot of patients end up with some cognitive slowing and cognitive problems. In those patients, we find their sleep looks older than it ought to by a couple of years.
Similarly, we recently found that the brain activity in patients with dementia looks older. If you have advanced dementia, it looks older than if you have early dementia.
Interestingly, people who have come in worried they're not as sharp as they used to be but are told by the doctor that they don't have a disease and don't get a diagnosis of dementia, these patients do have elevated brain age relative to the healthy normal. This suggests that this may be a way to get early signals of problems and hopefully intervene.
What does the Sleep Brain Age Index tell us about our chronological versus biological age and our risk of dementia?
They are related concepts. The BAI tell you your biological age through the lens of sleep. It's the measure of how much your biological age exceeds, or is under, your chronological age. Excess biological age correlates with risk for dementia or brain health problems more generally.
Along the lines of "outcome-driven AI," we're developing what we hope will be a more specific kind of predictor for risk of dementia, stroke and other kinds of brain health outcomes.
We thought: Wouldn't it be better if you could optimize our BAI algorithm directly to predict these outcomes? But this has some challenges from a technical standpoint.
For example, we know everybody's age who comes in to get a sleep study. Predicting a target like dementia is much more important and interesting than predicting someone's age, but we obviously don't have brain tissue samples or a formal assessment of cognitive function for most patients, so we don't have direct information. So, the amount of data to learn from is less.
That said, we're able to leverage our ability to measure biological brain age in every patient with the data we have from the smaller number of patients who come in with cognitive problems to create an algorithm that measures the risk of eventually developing dementia.
Using a combination of machine-learning techniques we've used before as well as survival analysis techniques, we can now tell you that based on your sleep, your risk is two times or four times elevated relative to baseline.
This new algorithm isn't published yet, but it's actually working and looking remarkably good!